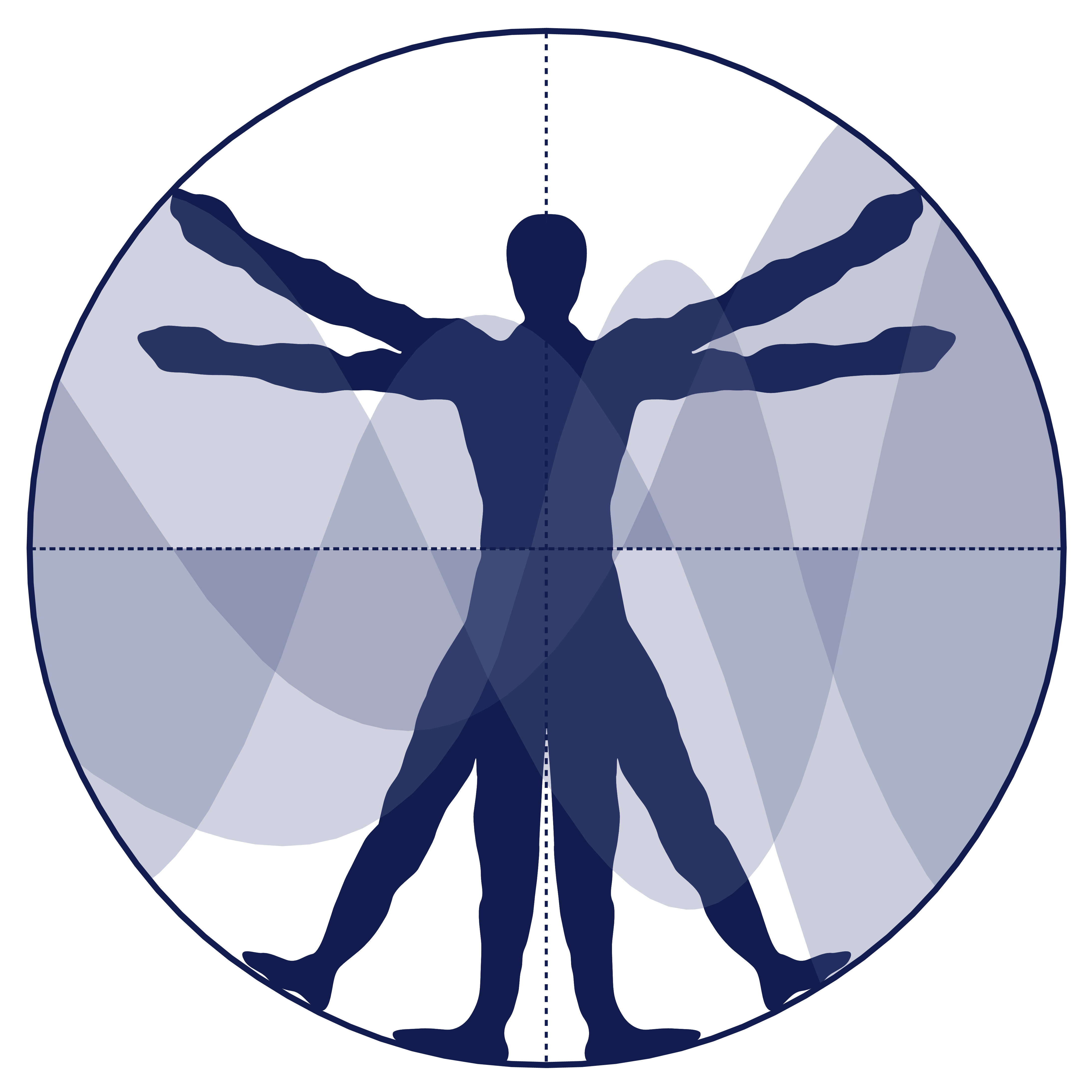
The majority of computational tasks in the field of medical physics cover the range of modeling, reconstruction, optimization and AI – all of which are prime candidates to benefit from the utilization of quantum computing. Quantum computing can solve complex problems that either cannot be resolved by classical computers or can only be approximated with utilizing high-performance computing. In contrast, quantum advantage may come in various forms: quantum speedup, more precise calculations, or simplified algorithmic complexity compared to classical algorithms. Any of these advantages alone or combined can have profound implications for medical science and precision medicine.
Quantum computing is a rapidly developing field. Nevertheless, as of 2022, the majority of quantum computing research worldwide has been focusing on quantum hardware developments. While numerous quantum algorithms have been proposed on a theoretical level, their practical experimentation in real clinical settings is underrepresented. The goal is to exploit the potential of quantum computing in clinically-relevant fields such as imaging, image analysis and Artificial Intelligence (AI) to increase diagnostic accuracy and to support precision medicine throughout the following objectives:
- Native Quantum Computing: To propose novel image reconstruction and analysis approaches in clinically-relevant fields utilizing quantum computing approaches and noisy intermediate scale quantum (NISQ) computers.
- Classic-to-Quantum Transitioning: To consolidate and transition existing classical computing methodologies to the quantum computing domain in order to make them adoptable in small-qubit NISQs.
- Clinical Adoption: To investigate novel quantum circuit visualization principles for supporting future clinical adoption and interpretation of quantum computing applications in clinical routine.
Research Area Representative
Laszlo Papp
P +43 (0)1 40400-72350
More about this research area
Quantum Photon-Tissue Interaction Modeling
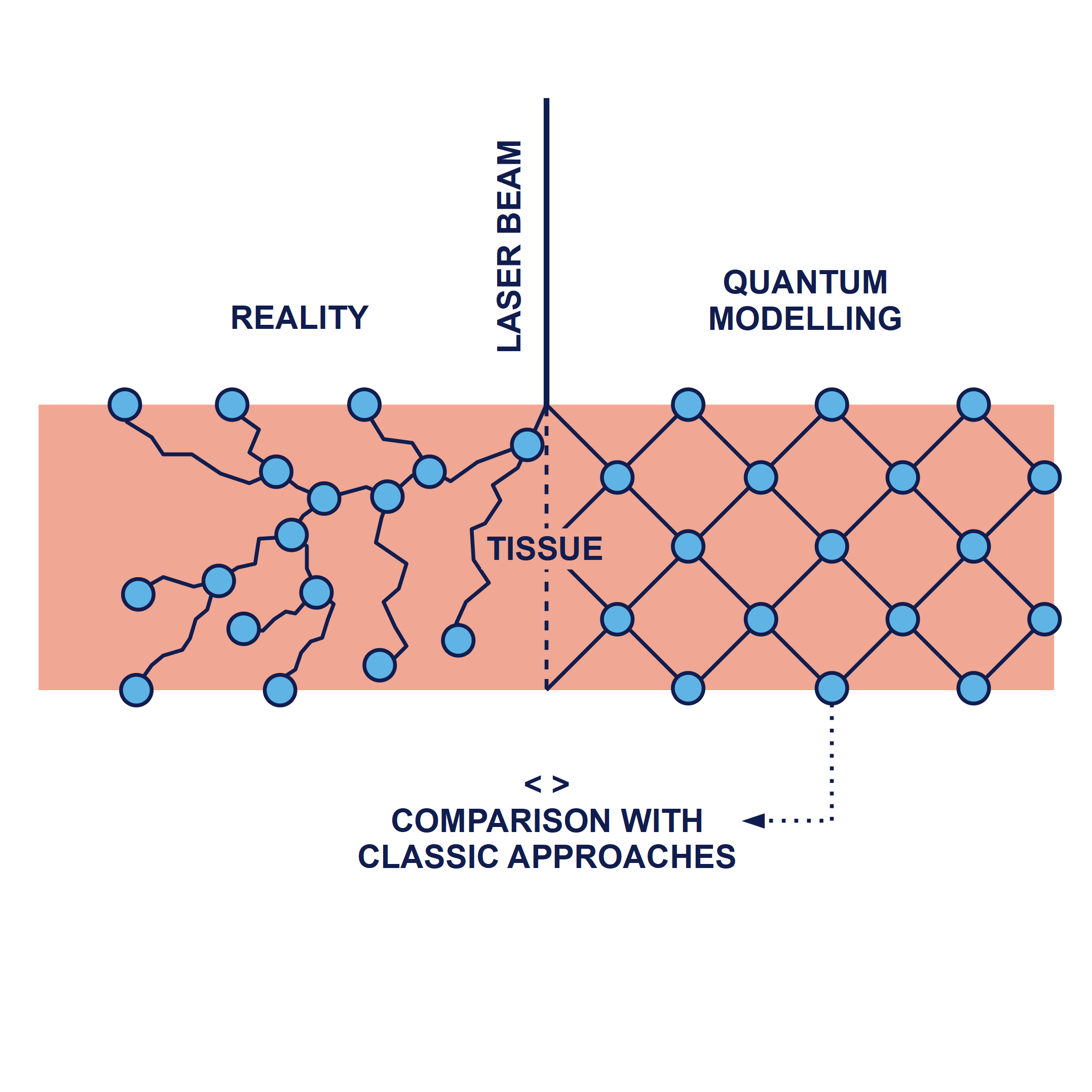
Photon-tissue interaction is a phenomenon which allows to acquire medical images such as optical coherence topography (OCT), computed tomography (CT) or positron emission tomography (PET). Medical imaging research – including research being conducted at our center – heavily relies on acquiring, reconstruction and analyzing medical images in order to characterize diseases in clinically-relevant imaging cohorts. This project aims to build up the fundamental building blocks of photon-tissue interaction for medical image reconstruction in the quantum domain by investigating various approaches such as quantum walk, optimization as well as solving heat equations. We currently focus on OCT imaging examples, since it can deliver proof-of-concept with small data (high resolution but in a small field of view) and in 2D. Findings of this project will be continuously adopted to 3D imaging.
Quantum Radiomics and AI
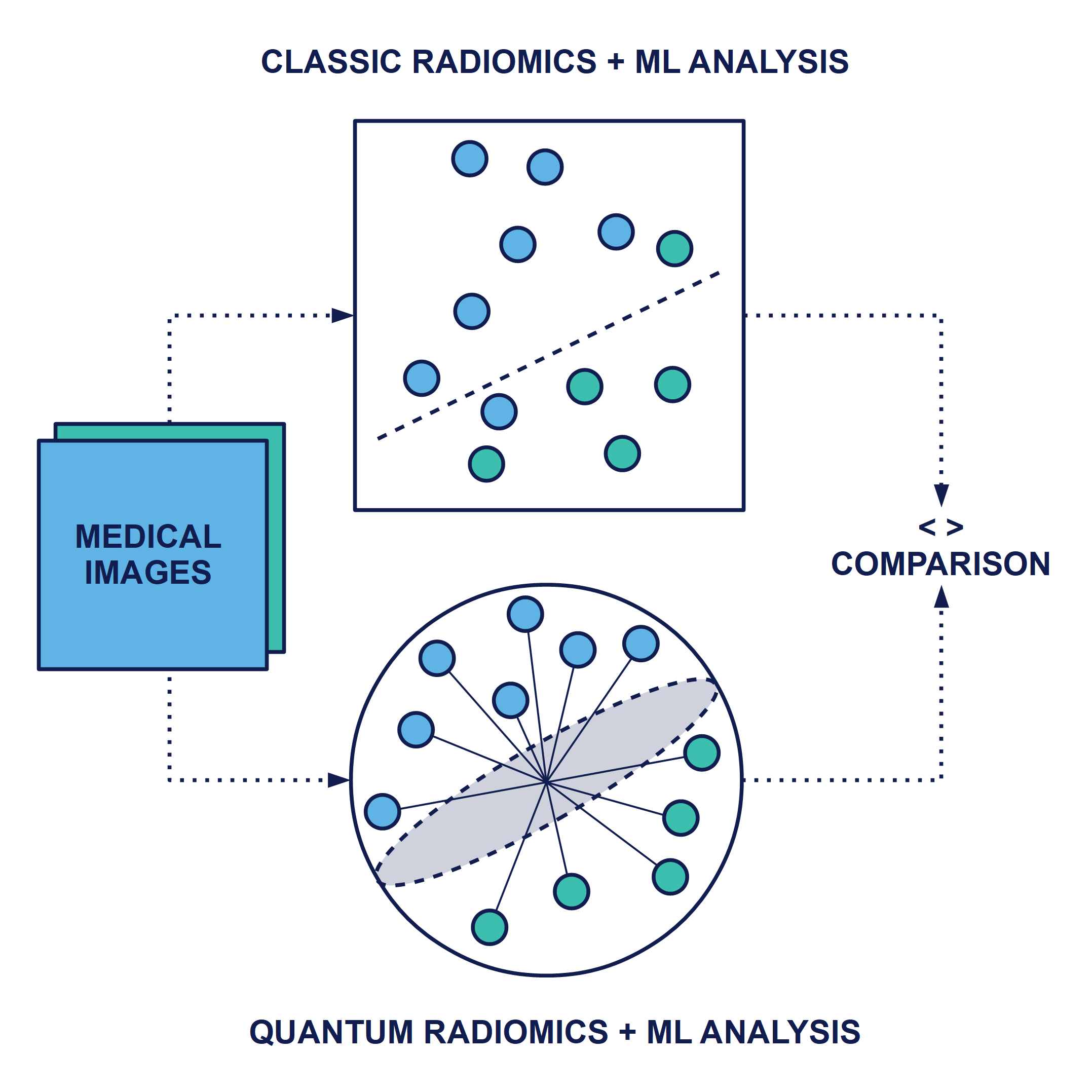
Radiomics is a process to extract various numerical features from medical images in order to analyte and predict form them by machine learning (ML) approaches. Deep learning approaches are considered superior to radiomics; however, they require large amounts of training data which is mostly not available. Previously proposed approaches imply that quantum feature extraction is possible by encoded medical images in the quantum domain, and that these quantum features have the potential to act as surrogates of both radiomic and deep features in one integrated, and simplified quantum circuit. Existing quantum machine learning solutions have been proven to bring benefit compared to their classical counterparts in various real-life datasets, including medical data, however, they still require classical data to be encoded before analysis. A native quantum feature extraction approach combined with native quantum machine learning can provide comparable or superior predictive performance compared to classic radiomics/deep learning and ML. Therefore, this project aims to investigate the added value of quantum radiomics + quantum ML in clinically-relevant imaging datasets.